Working Toward Responsible AI That Serves Society
Significant advancements in artificial intelligence (AI) are accelerating the establishment of more efficient decision-making processes. Nevertheless, entrusting responsibility for choices to a machine or program raises major ethical questions.
Whether dealing with the reproduction of biases present in datasets or the social acceptability of decisions, there are technological, legal, societal, and philosophical questions alike. Some actors are therefore committed to so-called explainable AI technology, which aims to help human experts understand the criteria underpinning the choices made by AI.
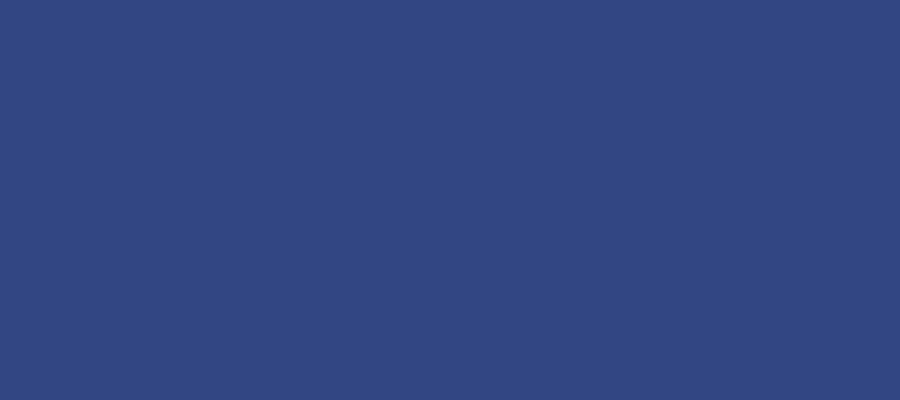
Applications
The high-level scientific research in mathematics and computer science at Dauphine-PSL is a core component of multidisciplinary approaches involving computer science, mathematical science, humanities, and social science.
The first step is to examine and predict the impact of these algorithms on public and private organizations, establish new modes of governance, and use interdisciplinary dialogue to design algorithms that naturally meet democratic requirements (lack of bias, respect of privacy, explainability, safety, and social acceptability).
Our Research in the University's Labs
Modern AI raises ethical questions regarding privacy, data manipulation, and the explainability of models and results, as well as equity and social responsibility of algorithms.
At the cutting edge of research in these fields, our activities encompass safe and reliable machine learning, the social responsibility of algorithms, and the characterization of ethical (prescriptive) rules and their incorporation into automated decision-making mechanisms.
Our Research by Topic
Reliable Machine Learning
The MILES project team brings together researchers in theoretical computer science, applied mathematics, and game theory.
Their research covers the entire field of machine learning, ranging from theoretical foundations to applications.
- Trustworthy machine learning: adversarial robustness; models preserving confidentiality (e.g. differential privacy); equity in machine learning
- Explainable and interpretable AI: causal inference; parsimonious models
- Compact and energy-efficient deep neural networks
Applications: Health Care – Games – Robotics – Computer Vision – Arts and Humanities
Social Responsibility of Algorithms
In 2017, Dauphine-PSL and its partners at DIMACS (the Center for Discrete Mathematics and Theoretical Computer Science, USA) and the 3AI Institute (Australia) launched a series of global conferences dealing with the social responsibility of algorithms.
The goal of these events, apart from precisely defining research questions with an interdisciplinary approach, is to produce bodies of knowledge that can be used to inform researchers, developers, and decision makers.
Ethics, Social Norms, and Equitable Decision-Making
Computational social choice is a field at the intersection of economics, game theory, and computer science dealing with the design and analysis of collective decision-making mechanisms (e.g. equitable resource sharing) with respect to the axiomatic characterization of decision-making mechanisms and the impact, on their feasibility, of their algorithmic complexity, information needs, and vulnerability to strategic behaviors.
Ethical questions (equality, equity, etc.) find in computational social choice the tools needed to study them, particularly when the questions are raised in terms of automated decision-making as in machine learning.
Through LAMSADE, Dauphine-PSL has a research tradition firmly anchored in the axiomatic characterization of decision-making and is home to a school of global renown in the field of computational social choice.
Our Researchers
Alexandre Allauzen, Jamal Atif, Tristan Cazenave, Yann Chevaleyre, Jérôme Lang, Rida Laraki, Benjamin Negrevergne, Fabrice Rossi, Clément Royer, Florian Yger, Alexis Tsoukias, Thierry Kirat
Published Research
- Interdisciplinary Workshop SRA 2019 – Social Responsibility of Algorithms
- Interdisciplinary Workshop SRA 2017 – Social Responsibility of Algorithms
- Pinot, R., Meunier, L., Araujo, A., Kashima, H., Yger, F., Gouy-Pailler, C., Atif, J. Theoretical evidence for adversarial robustness through randomization. In Advances in Neural Information Processing Systems (pp. 11838-11848) (2019).
- Airiau S., Aziz H., Caragiannis I., Kruger J., Lang J., Peters D. Portioning Using Ordinal Preferences: Fairness and Efficiency. Peters IJCAI 2019 : 11-17
- Aziz H., Bouveret S., Caragiannis I., Giagkousi I., Lang J. Knowledge, Fairness, and Social Constraints. AAAI 2018 : 4638-4645
- Beynier A., Chevaleyre Y., Gourvès L., Harutyunyan A., Lesca J., Maudet N., Wilczynski A. Local envy-freeness in house allocation problems. Auton. Agents Multi Agent Syst. 33(5) : 591-627 (2019)
- Yamane I., Yger F., Atif J., Sugiyama, M. Uplift modeling from separate labels. In Advances in Neural Information Processing Systems (pp. 9927-9937) (2018).
- Clertant M., Sokolovska N., Chevaleyre Y., Hanczar, B. Interpretable Cascade Classifiers with Abstention. In The 22nd International Conference on Artificial Intelligence and Statistics (pp. 2312-2320) (2019, April)
- Pinot R., Morvan A., Yger F., Gouy-Pailler C., Atif J. Graph-based Clustering under Differential Privacy. UAI 2018 : 329-338